Transferring Dextrous Surgical Skills among Robots
Building a real to real and simulation to real framework for knowledge transfer among robots.
Short response times are critical in austere environements such as a battlefield. Equpping robots with autonomous decision making capability, especially in critical tasks such as surgery, helps overcome communiction delays and reduces the reliance on expert staff. However, obtaining surgical data for austere environements can be dfficult. Hence, in this work, we present the Dexterous Surgical Skill (DESK) database for knowledge transfer among simulated and real robots, as shown in Figure below.
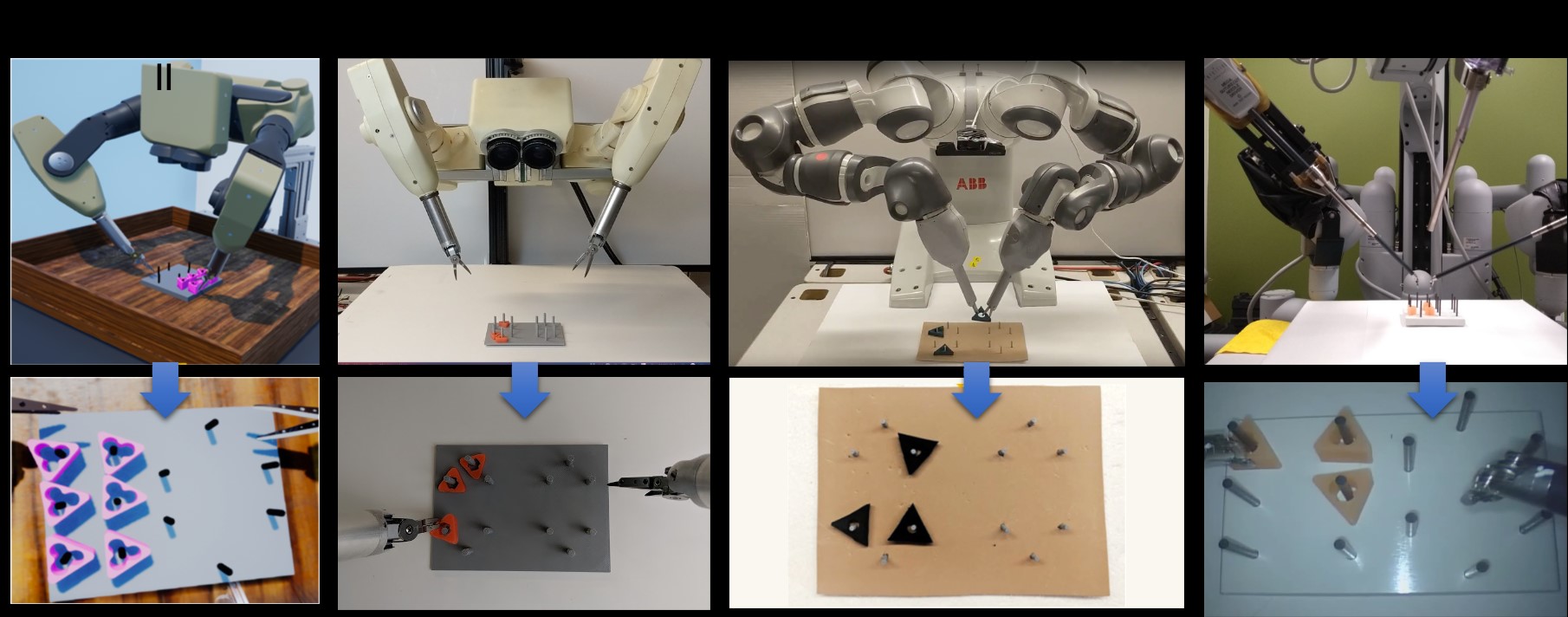
The simulated and real robots included in the DESK dataset for the peg transfer task, including the simulated Taurus robot, Taurus robot, Yumi robot, and the DaVinci robot.
We also present a unique machine learning-based transfer learning framework that extracts unique features for transferring kinemtic knowledge for each primitive motion among robots. The framework operates in the frequency domain, removes low-grade noise, and enables the robot to learn the unique distribution of each surgeme. Laproscopic surgery includes some key primitive motions also known as surgemes. We were able to achieve a maximum accuracy of 97.5% with this framework for transferring knowledge to the DaVinci Surgical systems.